Machine learning is accelerating the translation of biological and biomedical data to advance the detection, diagnosis, treatment, and prevention of diseases. However, the unprecedented scale and complexity of large-scale biomedical data have presented critical computational bottlenecks requiring new concepts and enabling tools. To address the challenging problems in the emerging biomedical and health applications, we proposed multiple novel nonlinear machine learning models with theoretical foundations for scalable nonlinear association study, interactive feature detection, multi-dimensional data integration, longitudinal feature learning, etc. Meanwhile, to deal with the big data computations, we proposed new asynchronous distributed stochastic gradient and coordinate descent methods for efficiently solving convex and non-convex problems, and also parallelized the deep learning optimization algorithms with layer-wise model parallelism and the first theoretical convergence guarantee.
We applied our new large-scale nonlinear machine learning models to analyze the multi-modal and longitudinal neuroimaging and genome-wide array data in Imaging Genetics and discover the phenotypic and genotypic biomarkers to characterize the neurodegenerative process in the progression of Alzheimer’s disease and other complex brain disorders. We also utilized our new machine learning models to analyze the Electronic Medical Records (EMR) for predicting the heart failure patients’ readmission and drug side effects, identify the histopathological image markers and the multi-dimensional cancer genomic biomarkers in The Cancer Genome Atlas (TCGA) for precision medicine, and guide design of nanoparticle synthesis in Materials Genome research, and detect the DTI and fMRI based brain circuitry patterns in Human Connectome.
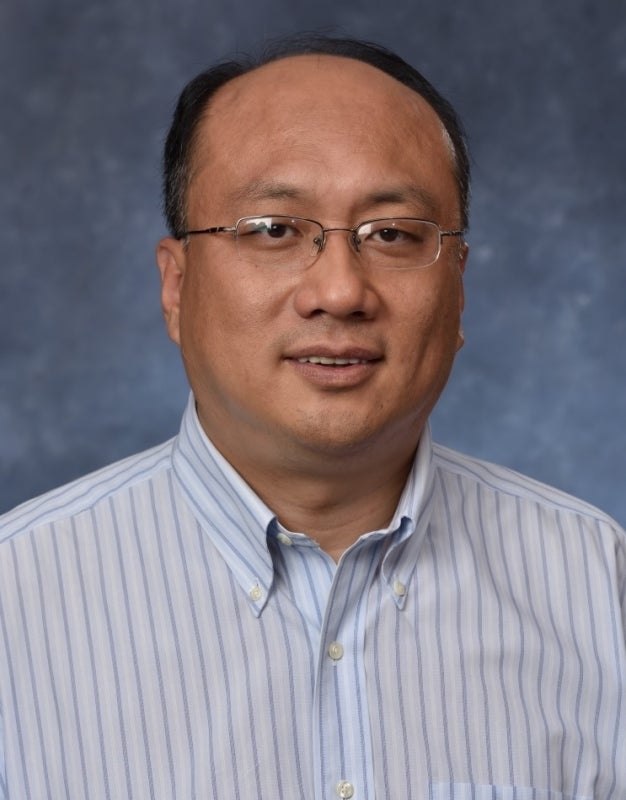
Dr. Heng Huang is John A. Jurenko Endowed Professor in Electrical and Computer Engineering at University of Pittsburgh, and also Professor in Biomedical Informatics at University of Pittsburgh Medical Center. Dr. Huang received the PhD degree in Computer Science at Dartmouth College. His research areas include machine learning, big data mining, medical image analysis, system biology, smart and connected health, neuroinformatics, and precision medicine. Dr. Huang has published more than 220 papers in top-tier conferences and many papers in premium journals, such as NeurIPS, ICML, KDD, RECOMB, ISMB, ICCV, CVPR, IJCAI, AAAI, IPMI, MICCAI, Bioinformatics, Medical Image Analysis, Neurobiology of Aging, IEEE TMI, TKDE, TNNLS, etc. Based on csrankings.org, for the last ten years, Dr. Huang is ranked 3rd among researchers who published most top computer science conference papers. As PI, Dr. Huang currently is leading NIH R01 and multiple NSF funded projects on machine learning, neuroimaging, big data computation, precision medicine, electronic medical record data analysis and privacy-preserving, smart healthcare, and cyber physical system. Dr. Huang serves as the Program Chair of ACM SIGKDD Conference 2020.