Deciphering how the brain works requires new methods for recording and interpreting activity from large neural populations at single-neuron resolution. In this talk I will focus on one important and widely used optical neural recording modality: two-photon microscopy (TPM). While multiphoton imaging of brain activity has allowed for large-scale recordings of hundreds-to-thousands of neurons, many challenges remain, including scaling of imaged volume size, robustness of neural activity inference from fluorescence videos, analysis of non-somatic data (e.g., dendritic, widefield, and synaptic imaging), and validation of 2P methods. I will present a sequence of papers that addresses these challenges. Specifically I will discuss 1) a volumetric imaging technique based on computational imaging using stereoscopic measurements, 2) a robust estimator of calcium activity based on sparse modeling of missing regressors, 3) a graph-signal processing based approach to generalized analysis across morphologies, 4) a deep-learning approach to synnaptic image denoising, and finally 5) a biophysical simulation suite designed to enable large-scale assessment of calcium imaging methods. These advances, taken together, provide a much expanded toolbox for obtaining and analyzing neural data at scale.
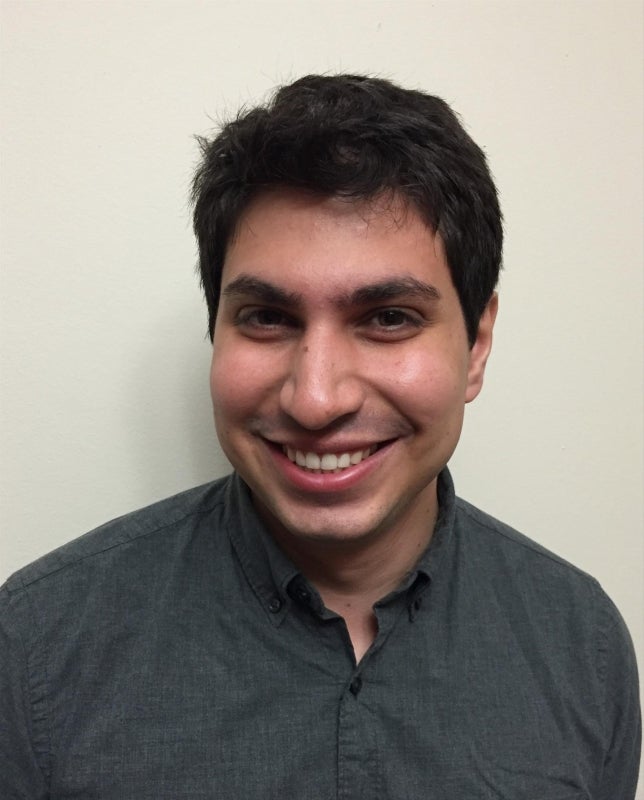
Adam Charles is an Assistant Professor at the Department of Biomedical Engineering at Johns Hopkins University, with affiliations with the Center for Imaging Science, the Mathematical Institute for Data Science, the Kavli Neuroscience Discovery Institute, and the Department of Neuroscience. His research focuses on the acquisition and interpretation of neural data, including neural imaging technologies, inference and tracking of sparse and structured signals, and mathematical modeling of neural networks. He received both a B.E. and M.E in Electrical and Computer Engineering in 2009 from The Cooper Union in New York City. He received his Ph.D. in Electrical and Computer Engineering in 2015 at The Georgia Institute of Technology, where his research was awarded a Sigma Xi Best Doctoral Thesis award as well as an Electrical and Computer Engineering Research Excellence award. Post-graduation, Adam joined the Princeton Neuroscience Institute, as a post-doctoral fellow, working on computational neuroscience and data analysis methods.