Advances in digital pathology and artificial intelligence have presented the potential to build assistive tools for objective diagnosis, prognosis and therapeutic-response and resistance prediction. In this talk we will discuss our work on: (1) Data-efficient methods for weakly-supervised whole slide classification with examples in cancer diagnosis and subtyping (Nature BME, 2021), identifying origins for cancers of unknown primary (Nature, 2021) and allograft rejection (Nature Medicine, 2022) (2) Discovering integrative histology-genomic prognostic markers via interpretable multimodal deep learning (Cancer Cell, 2022; CVPR, 2024; ICML, 2024). (3) Building unimodal and multimodal foundation models for pathology, contrasting with language and genomics (Nature Medicine, 2024a, Nature Medicine 2024b, CVPR, 2023). (4) Developing a universal multimodal generative co-pilot and chatbot for pathology (Nature, 2024). (5) 3D Computational Pathology (Cell, 2024) (6) Bias and fairness in computational pathology algorithms (Nature Medicine, 2024).
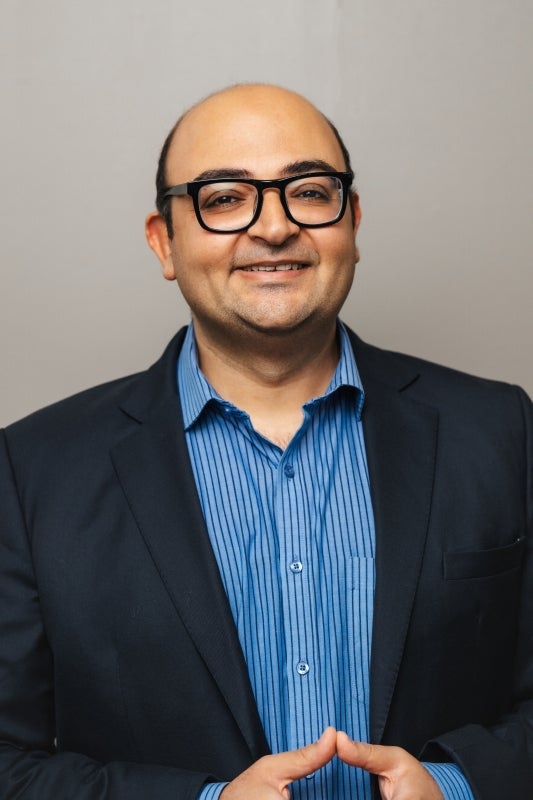
Dr. Mahmood is an Associate Professor at Harvard Medical School and the Division of Computational Pathology at the Brigham and Women's Hospital and Massachusetts General Hospital. He received his Ph.D. in Biomedical Imaging from the Okinawa Institute of Science and Technology, Japan and was a postdoctoral fellow at the department of biomedical engineering at Johns Hopkins University. Dr. Mahmood’s research focuses on developing generative and multimodal AI for pathology applications. Dr. Mahmood is a full member of the Dana-Farber Cancer Institute / Harvard Cancer Center ; an Associate Member of the Broad Institute of Harvard and MIT, and a member of the Harvard Bioinformatics and Integrative Genomics (BIG) faculty. Learn more about his research lab at www.mahmoodlab.org